How to solve these 3 common chatbot implementation challenges
Posted: Thu Dec 05, 2024 5:58 am
The chatbot market – set to be worth $9.4 billion as soon as 2024 – is seeing major growth as adoption grows and organizations realize the tangible business benefits of both AI chatbots and simple keyword chatbots.
But even with the easiest to use chatbot building platforms, building a chatbot doesn’t come without a few common challenges.
Here we’ll take look at some of the common chatbot implementation challenges – and how to solve them.
Chatbot implementation challenge 1: measuring value
There are compelling business benefits to adding a chatbot to your customer service mix. But all too often, businesses build a chatbot for the sake of it.
This means they’ll invest in designing, building and deploying a chatbot without clearly defining their business need or objective the chatbot must support.
Without defining these crucial first steps, businesses will struggle to measure the value their chatbot generates.
This measurable framework needs to be baked in from the start.
How to measure chatbot value
Start with what you know.
Research which customer support enquiries your team most commonly handles, and equip your chatbot to deal with these questions.
Doing this allows you to gradually move repetitive tasks from your customer support team over to your chatbot.
This helps you achieve two important business benefits:
customers receive instant support, resulting in higher customer satisfaction
customer support agents receive fewer repetitive queries, for improved agent efficiency and job satisfaction
Once you equip your chatbot to handle low-value, high-volume enquiries, start gradually introducing progressively more complex customer support tasks.
Before you know it, your chatbot will be supporting the teams business owner database that support your customers – which brings the most value.
You don’t launch a chatbot simply because your competitor did – you launch it to help you drive efficiencies in your customer service and get to your goals faster. Check out our related post on chatbot success metrics to learn more.
Chatbot implementation challenge 2: understanding customer intents
We differentiate two main chatbot types, depending on how users interact with them.
These are simpler keyword, and more complex conversational AI chatbots.
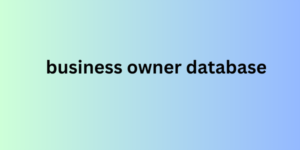
Tip: learn more about keyword and intent-based chatbots.
With simpler keyword bots, user intents are easy to figure out. You serve them a list of options or keywords, and the user selects from this range of options. Easy.
But when it comes to AI (or intent-based) chatbots, things can get a lot more complex.
Getting machines to seamlessly interact with humans used to be massive challenge.
But with systems like Open AI’s ChatGPT-3, it’s simpler than ever for humans and machines to have actual conversations.
However, there’s still a bit of an uncanny valley to cross in order to facilitate natural conversations between your customers and chatbot.
Here’s how:
Programming a chatbot to understand common customer queries
To program a chatbot to talk to your customers, you first need to know what your customers want to talk about.
Find out what are the most frequently asked questions your customers are asking.
Next, research phrase variations of these most common questions.
Protip: Experts recommend training chatbots to understand at least fifty phrase variations.
This is no small task, of course – which is why the best chatbot platforms have experts on hand to help their clients develop phrase variation databases.
Chatbot implementation challenge 3: seamless agent takeover
Chatbots are great, but they don’t have superpowers. While they can handle your most common customer interactions, there are limits to what they can handle.
But even with the easiest to use chatbot building platforms, building a chatbot doesn’t come without a few common challenges.
Here we’ll take look at some of the common chatbot implementation challenges – and how to solve them.
Chatbot implementation challenge 1: measuring value
There are compelling business benefits to adding a chatbot to your customer service mix. But all too often, businesses build a chatbot for the sake of it.
This means they’ll invest in designing, building and deploying a chatbot without clearly defining their business need or objective the chatbot must support.
Without defining these crucial first steps, businesses will struggle to measure the value their chatbot generates.
This measurable framework needs to be baked in from the start.
How to measure chatbot value
Start with what you know.
Research which customer support enquiries your team most commonly handles, and equip your chatbot to deal with these questions.
Doing this allows you to gradually move repetitive tasks from your customer support team over to your chatbot.
This helps you achieve two important business benefits:
customers receive instant support, resulting in higher customer satisfaction
customer support agents receive fewer repetitive queries, for improved agent efficiency and job satisfaction
Once you equip your chatbot to handle low-value, high-volume enquiries, start gradually introducing progressively more complex customer support tasks.
Before you know it, your chatbot will be supporting the teams business owner database that support your customers – which brings the most value.
You don’t launch a chatbot simply because your competitor did – you launch it to help you drive efficiencies in your customer service and get to your goals faster. Check out our related post on chatbot success metrics to learn more.
Chatbot implementation challenge 2: understanding customer intents
We differentiate two main chatbot types, depending on how users interact with them.
These are simpler keyword, and more complex conversational AI chatbots.
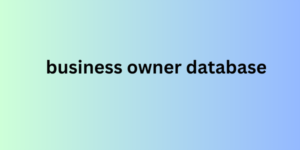
Tip: learn more about keyword and intent-based chatbots.
With simpler keyword bots, user intents are easy to figure out. You serve them a list of options or keywords, and the user selects from this range of options. Easy.
But when it comes to AI (or intent-based) chatbots, things can get a lot more complex.
Getting machines to seamlessly interact with humans used to be massive challenge.
But with systems like Open AI’s ChatGPT-3, it’s simpler than ever for humans and machines to have actual conversations.
However, there’s still a bit of an uncanny valley to cross in order to facilitate natural conversations between your customers and chatbot.
Here’s how:
Programming a chatbot to understand common customer queries
To program a chatbot to talk to your customers, you first need to know what your customers want to talk about.
Find out what are the most frequently asked questions your customers are asking.
Next, research phrase variations of these most common questions.
Protip: Experts recommend training chatbots to understand at least fifty phrase variations.
This is no small task, of course – which is why the best chatbot platforms have experts on hand to help their clients develop phrase variation databases.
Chatbot implementation challenge 3: seamless agent takeover
Chatbots are great, but they don’t have superpowers. While they can handle your most common customer interactions, there are limits to what they can handle.